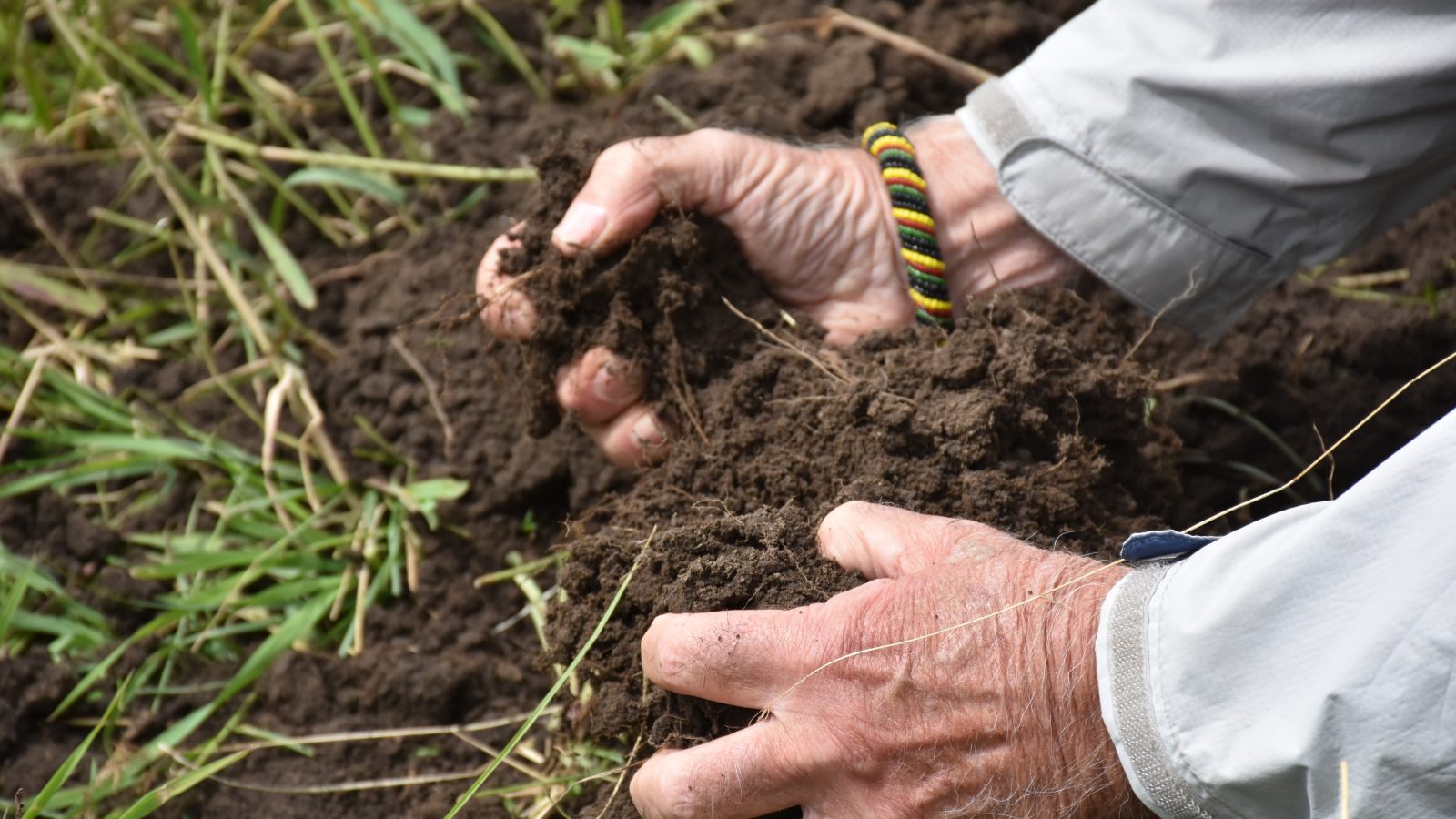
David Green, Executive Director, and Maite Caballero, Senior Researcher from The U.S. Sustainability Alliance, argue that AI technologies pave the way for healthier soils in agriculture.
The clock is ticking. More than 200,000 people are added to the world’s population daily, and they would all like to eat. Global agriculture needs to produce enough food to feed the 10 billion humans expected on our planet by 2050.
Already, farmers worldwide face daily challenges due to changes in temperatures, increasingly heavy precipitation, erosion, agricultural land losses, and unpredictable weather patterns. Critically, these events impact food security, with a projected reduction in most crops worldwide. According to a NASA-led scientific study, this includes maize production, which is set to decrease by 24% by 2030. (1)
It is becoming increasingly evident that healthy soils will be crucial for the world’s future food supply. In many croplands worldwide, even the ‘black gold’ earth of the American Midwest, the layer of fertile topsoil is thinning, making it more difficult to grow food. Conserving (and building) the fertility of our soils is the biggest challenge facing today’s agriculture. (2)
Artificial Intelligence and Creating Healthier Soils
Soil health is determined by organic matter content, porosity, nutrient content and pH, erosion resistance, water management, and biotic interactions (crop rotation and plant diversity). Assessing and evaluating farming practices is crucial to discern which are effective in restoring healthier soils and which are detrimental. Artificial Intelligence (AI) and Machine Learning (ML) are fast supplementing conventional approaches. Indeed, AI techniques that include machine learning are incredibly accurate in making soil texture predictions.
How Do AI and ML Techniques Work?
AI and ML techniques collect data from extensive soil sampling and historical data to create comprehensive predictive soil maps. Traditionally, soil health is determined through sieving, sedimentation, and soil analysis, which is time-consuming, sometimes inaccurate, and requires skilled professionals and specialist tools.
However, AI can process enormous amounts of data that can be linked to historical soil data to establish patterns and relationships and used to make predictions or classifications. Applying the AI model to new soil data can, for example, help predict nutrient levels, determine soil moisture, assess soil erosion risk, or classify soil types based on data.
When AI is combined with remote sensing technologies such as satellite imagery or airborne sensors, the information obtained is even more efficient and reliable. As stated in the study “AI and machine learning for soil analysis: an assessment of sustainable agricultural practices” (3), artificial intelligence can also process non-numerical data, such as images, videos, text, and voice data, with greater perfection than the human brain.
AI Technologies in Agriculture: The Benefits
By leveraging AI technologies, farmers and land managers can make data-driven decisions, optimize resource use, and implement practices that protect and enhance soil health while building a more resilient food production system.
There are many applications of AI in agriculture, including:
- Monitoring and digitizing soil data provides information on the soil’s ability to promote plant growth, helping farmers make informed decisions about water and nutrient use.
- Pest control, either by predicting pesticide application times or identifying potential pests that can damage the soil.
- Risk management and precision forecasting use algorithms to predict variables such as plant strain, soil data, preparation, irrigation, and supply chain management.
- Planning for the precise application of fertilizers, pesticides, and irrigation.
- Locating carbon sinks and increasing carbon capture in soil.
AI and soil studies abound in Europe and in the United States, where lessons from the 1930s Dust Bowl continue to focus the mind. The U.S. Department of Agriculture’s Natural Resources Conservation Service (USDA NRCS), for example, is funding a Worcester Polytechnic Institute study using an AI-based radar system to provide accurate measurements of root-zone soil moisture, which could increase irrigation efficiency by 50%. (4)
Likewise, The Missouri Partnership, a public-private economic development organization, has partnered with Climate FieldViewTM to provide farmers with data that will enable them to make real-time decisions on the farm and maximize their returns. (5)
Challenges for AI to Determine Soil Health
However, using AI to determine soil health is not without challenges. As with any innovation, AI technology is expensive and not easily accessible to smaller farms. Farmers and land managers need knowledge and expertise to handle and understand AI systems, which requires training and a considerable time investment. Also, managing, storing, and securing the data required to establish patterns and predictions may conflict with data privacy laws in some States, so legal and regulatory considerations must be carefully assessed.
U.S. stakeholders agree on the need to establish international collaboration and innovation, not just in terms of joint research but also regulatory cooperation and open sharing of scientific data.
The Future of AI in Agriculture
AI and machine learning techniques are emerging as game-changers in agriculture, and according to the latest Precedence Research report (6), their global importance is only set to grow – from a market estimated at $1.37 billion in 2022 to more than $11.13 billion by 2032 – paving the way for more sustainable soil management practices. To feed an ever-growing population in the years ahead, farmers will need innovative and accessible technologies, and AI is poised to play a pivotal role.
References
- Jägermeyr, J., Müller, C., Ruane, A.C. et al. Climate impacts on global agriculture emerge earlier in new generation of climate and crop models. Nat Food 2, 873–885 (2021). https://doi.org/10.1038/s43016-021-00400-y
- https://thesustainabilityalliance.us/the-answer-lies-in-the-soil/
- Awais, M., Naqvi, S.M.Z.A., Zhang, H. et al. AI and machine learning for soil analysis: an assessment of sustainable agricultural practices. Bioresour. Bioprocess. 10, 90 (2023). https://doi.org/10.1186/s40643-023-00710-y
- https://www.wpi.edu/news/seyed-zekavat-leads-11-million-project-develop-system-will-create-soil-moisture-maps-farmers
- https://www.missouripartnership.com/missouri-agtech-company-launches-partnership-bring-big-data-farmers/
- Artificial Intelligence in Agriculture Market (By Component: Hardware, Software, Services; By Technology: Machine Learning & Deep Learning, Predictive Analytics, Computer Vision; By Application: Precision Farming, Drone Analytics, Agriculture Robots, Livestock Monitoring, Others) – Global Industry Analysis, Size, Share, Growth, Trends, Regional Outlook, and Forecast 2023-2032.
This article first appeared on Open Access Government – a digital publication that provides an in-depth perspective on key public policy areas worldwide, including research and innovation, technology, government, the environment, and energy.